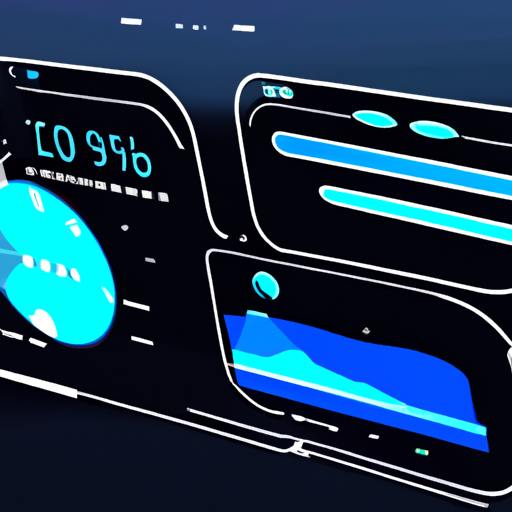
The Role of MAPE in Forecast Accuracy
The Importance of MAPE in Forecast Accuracy
When it comes to forecasting, accuracy is key. Businesses rely on accurate forecasts to make informed decisions about production, inventory, and sales. One commonly used metric to measure forecast accuracy is MAPE, which stands for Mean Absolute Percentage Error. MAPE is a valuable tool that helps businesses evaluate the effectiveness of their forecasting methods and make improvements where necessary.
So, what exactly is MAPE and why is it important? MAPE is a measure of the average percentage difference between the forecasted values and the actual values. It provides a clear picture of how well a forecast is performing by quantifying the magnitude of errors. By calculating the average percentage error, businesses can determine the overall accuracy of their forecasts and identify areas for improvement.
One of the key benefits of using MAPE is that it allows businesses to compare the accuracy of different forecasting methods. For example, if a company is using two different forecasting models, they can calculate the MAPE for each model and determine which one is more accurate. This information can then be used to make informed decisions about which forecasting method to use in the future.
Another important aspect of MAPE is that it provides a standardized measure of forecast accuracy. This means that businesses can compare their forecast accuracy to industry benchmarks or to other companies in the same industry. By benchmarking their performance against others, businesses can gain valuable insights into how well they are doing and identify areas where they may be falling behind.
In addition to benchmarking, MAPE can also be used to track forecast accuracy over time. By calculating MAPE for each forecast period, businesses can identify trends and patterns in their forecasting performance. For example, if the MAPE is consistently high for a particular product or market segment, it may indicate that there are underlying issues with the forecasting process that need to be addressed.
Furthermore, MAPE can help businesses identify and address bias in their forecasts. Bias occurs when a forecast consistently overestimates or underestimates the actual values. By calculating MAPE, businesses can determine if there is any bias in their forecasts and take corrective actions to improve accuracy. This could involve adjusting forecasting models, gathering more accurate data, or refining the forecasting process.
It is worth noting that while MAPE is a valuable tool for evaluating forecast accuracy, it does have some limitations. For instance, MAPE is sensitive to extreme values or outliers, which can skew the results. Additionally, MAPE does not provide any information about the direction of the errors, only the magnitude. Despite these limitations, MAPE remains a widely used and accepted metric for measuring forecast accuracy.
In conclusion, MAPE plays a crucial role in evaluating forecast accuracy. It provides businesses with a standardized measure to compare their performance to industry benchmarks and identify areas for improvement. By tracking MAPE over time, businesses can identify trends and patterns in their forecasting performance and take corrective actions as needed. While MAPE has its limitations, it remains an essential tool for businesses looking to make accurate forecasts and make informed decisions.
Key Factors Influencing MAPE in Forecasting
Forecast accuracy is a crucial aspect of any business’s success. Accurate forecasts help companies make informed decisions, allocate resources effectively, and meet customer demands. One widely used metric to measure forecast accuracy is the Mean Absolute Percentage Error (MAPE). MAPE is a simple yet powerful tool that provides valuable insights into the accuracy of forecasts. In this article, we will explore the key factors that influence MAPE in forecasting.
One of the primary factors that affect MAPE is the quality of historical data. Accurate forecasts heavily rely on historical data, as it provides a basis for predicting future trends. If the historical data is incomplete, inconsistent, or contains outliers, it can significantly impact the accuracy of forecasts. Therefore, it is crucial for businesses to ensure that their historical data is clean, reliable, and representative of the actual demand patterns.
Another factor that influences MAPE is the forecasting method used. Different forecasting methods have varying levels of accuracy, and it is essential to choose the most appropriate method for the specific business context. For instance, time series forecasting methods, such as exponential smoothing or ARIMA, are commonly used for forecasting demand patterns with a strong historical trend. On the other hand, causal forecasting methods, such as regression analysis, are more suitable for predicting demand based on external factors like marketing campaigns or economic indicators. Selecting the right forecasting method can significantly improve the accuracy of forecasts and consequently reduce MAPE.
The level of aggregation is also a critical factor in determining MAPE. Aggregation refers to the level at which the forecasts are made, such as SKU level, product category level, or overall company level. Forecasting at a higher level of aggregation tends to result in lower MAPE, as it smooths out the variations in demand. However, forecasting at a more granular level can provide more accurate insights into specific product demands. Businesses need to strike a balance between the level of aggregation and the desired accuracy level to optimize their forecasting process.
The forecasting horizon is another factor that affects MAPE. The forecasting horizon refers to the time period for which the forecasts are made, such as daily, weekly, or monthly. Generally, shorter forecasting horizons tend to have lower MAPE, as they are closer to the actual demand. However, shorter forecasting horizons may not be suitable for all businesses, especially those with longer lead times or seasonal demand patterns. It is crucial to consider the nature of the business and the specific requirements when determining the forecasting horizon.
Lastly, the availability of real-time data can significantly impact MAPE. Real-time data provides up-to-date information on market trends, customer behavior, and other external factors that can influence demand. Incorporating real-time data into the forecasting process can improve the accuracy of forecasts and reduce MAPE. However, it is essential to have robust data collection and analysis systems in place to ensure the timely availability and accuracy of real-time data.
In conclusion, MAPE is a vital metric for measuring forecast accuracy. Several key factors influence MAPE in forecasting, including the quality of historical data, the forecasting method used, the level of aggregation, the forecasting horizon, and the availability of real-time data. By understanding and addressing these factors, businesses can improve the accuracy of their forecasts, make better-informed decisions, and ultimately enhance their overall performance.
Strategies for Improving MAPE in Forecast Accuracy
The Importance of MAPE in Forecast Accuracy
Forecast accuracy is crucial for businesses to make informed decisions and plan for the future. One commonly used metric to measure forecast accuracy is MAPE, which stands for Mean Absolute Percentage Error. MAPE calculates the average percentage difference between the forecasted values and the actual values. It provides a clear picture of how accurate the forecasts are and helps businesses identify areas for improvement.
Improving MAPE in forecast accuracy is essential for businesses to minimize errors and make more reliable predictions. By reducing MAPE, businesses can increase their confidence in their forecasts and make better-informed decisions. There are several strategies that businesses can employ to improve MAPE and enhance their forecast accuracy.
One strategy is to gather more accurate and reliable data. The quality of the data used for forecasting directly impacts the accuracy of the forecasts. Businesses should ensure that they collect data from reliable sources and use appropriate methods to clean and validate the data. By improving the quality of the data, businesses can reduce errors and improve the accuracy of their forecasts.
Another strategy is to use advanced forecasting techniques. Traditional forecasting methods may not always be the most accurate or efficient. Businesses can explore advanced techniques such as time series analysis, regression analysis, or machine learning algorithms to improve their forecasts. These techniques can help identify patterns, trends, and relationships in the data that may not be apparent with traditional methods. By leveraging advanced techniques, businesses can make more accurate predictions and reduce MAPE.
Collaboration and communication are also crucial for improving MAPE in forecast accuracy. Different departments within a business often have valuable insights and information that can contribute to more accurate forecasts. By fostering collaboration and open communication, businesses can gather diverse perspectives and incorporate them into their forecasting process. This can help identify potential biases, uncover hidden factors, and improve the overall accuracy of the forecasts.
Regular monitoring and evaluation of forecasts are essential for improving MAPE. Businesses should continuously track the accuracy of their forecasts and compare them to the actual outcomes. This allows them to identify any discrepancies and adjust their forecasting methods accordingly. By regularly evaluating the forecasts, businesses can learn from their mistakes, refine their techniques, and improve the accuracy of their future predictions.
Lastly, businesses should consider incorporating feedback loops into their forecasting process. Feedback loops involve collecting feedback from customers, sales teams, or other stakeholders to validate and refine the forecasts. By incorporating feedback, businesses can gain valuable insights into the accuracy of their forecasts and make necessary adjustments. This iterative process can help reduce errors, improve MAPE, and enhance forecast accuracy over time.
In conclusion, MAPE is a crucial metric for measuring forecast accuracy. Improving MAPE is essential for businesses to make reliable predictions and make informed decisions. By employing strategies such as gathering accurate data, using advanced techniques, fostering collaboration, monitoring and evaluating forecasts, and incorporating feedback loops, businesses can enhance their forecast accuracy and reduce MAPE. By continuously striving for improvement, businesses can increase their confidence in their forecasts and gain a competitive edge in the market.